In a brief diversion from the simecol dynamic system models, which I promise to resume in the future, I'll start another series of entertaining posts about solving mathematical/logic puzzles using R, of which this post you're reading is the first. This time around, we'll take a crack at the Problem of the Five Sailors, One Monkey and a Pile of Coconuts. Read on to find out what this is all about.
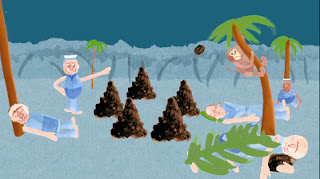
The Puzzle
This problem first appeared in the Saturday Evening Post in the 1920's and was later popularized by Martin Gardner
. It goes as follows:
Five sailors arrive at a deserted island that has only coconuts and one monkey. After laboriously collecting all the coconuts into one big pile, the sailors all agree to divide up the coconuts into equal shares the next morning. However, in the middle of the night a sailor wakes up and, not trusting the others, decides to give the monkey one coconut (to buy his silence?) and takes away his 1/5 share of the coconuts, leaving the rest in the pile. The other 4 sailors wake up at different time intervals and each does the same thing: give one coconut to the monkey and stash away his 1/5 share of the coconuts that remain in the pile. In the morning they divide what is left of the pile into equal shares and there is still one coconut left for the monkey. How many coconuts were in the pile the day before?
Readers in the 1920's did not have the benefit of Google or the Internet so after racking their brains for a while, many gave up in frustration and soon, the premises of the newspaper were flooded by an angry mob bearing pitchforks and torches, demanding to know the answer1. Now, before you fire up the Google search engine for the answer to this conundrum, let's first try a...
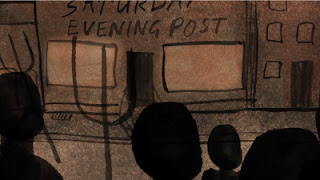
Brute-force solution
By brute-force, I really do mean brute as opposed to smart. It would seem that most people think mathematicians arrive at sophisticated answers directly in one step. Not me - I'm a dumb one so I usually try to explore the problem situation and try a little brute-force searching first. I don't actually have to do the computations myself, that's what R is for. Only after I understand the problem more thoroughly do I attempt to tackle it using more formal mathematical artifices.2
Our brute-force strategy will be to try out successive integer values for the size of the coconut pile to search for one where we will be able to deal out the coconut pile to the 5 sailors and the monkey in the conditions described by the problem. For each candidate integer value, we will simulate each of the five sailors taking their shares and giving one to the monkey, and in the end, dividing the pile into five equal shares plus one for the monkey. The key here is that the divisions in each step must be exact, since we can't deal with fractions of coconuts, otherwise the problem would be too easy, wouldn't it? Here is my brute-force solution script:
1 2 3 4 5 6 7 8 9 10 11 12 13 14 15 16 17 18 19 20 21 22 23 24 25 26 27 28 29 30 31 32 33 34 35 36 37 |
|
Number of coconuts in pile: 15621
Pile Sailor.s.share For.monkey
1 15621 3124 1
2 12496 2499 1
3 9996 1999 1
4 7996 1599 1
5 6396 1279 1
6 5116 1023 1
There it is - the pile originally had 15621 coconuts and there were 1023 coconuts for each sailor (and one for the monkey) at daybreak. The one thing that strikes us when we run the script above is how agonizingly slow it is. Since we initially have no idea how large the solution is, we brute-search in the first one million positive integers using the for loop in lines 27-293.
To check if an integer candidate is a solution to the problem, we simulate 6 partitionings (one for each of the five sailors and the final division of the coconuts done in the morning). Each partitioning consists of dividing the coconut pile into five equal parts and verifying that the remainder (integer modulo division) is one, which is the coconut for the monkey. If at some point in the process we do not get a remainder of 1, we will be exiting the verfication process, knowing that we could not perform all 6 iterations of it. This is what the code in lines 10-24 does.
Finally, note the output below the R script, indicating how many coconuts remain in the pile, the share for each sailor and the share for the monkey at each iteration. Could we have somehow guessed this would be the answer? Well, we could have ventured that since we were going to divide the pile by 5 six times, the answer would need to be divisible by \(5^6=15626\), this being our approximate answer. Of course, we would have known this could not be the answer to the puzzle because we had not taken into consideration the part about giving one coconut to the monkey at each step, which complicates things a great deal.
Diophantine equations to the rescue
Now that we know the answer to the problem, let's see if we can deduce it mathematically. Let \(C_0\) be the number of coconuts the five sailors originally collected. When the first sailor comes along in the middle of the night, he divides this pile into five equal parts and gives the remaining coconut to the monkey. So if \(S_1\) is his one fifth share of the coconuts, then the following equation holds: \(C_0=5S_1+1\). So when the next sailor comes along, there will be \(C_1=4S_1\) coconuts in the pile, the previous sailor having taken his share, plus one for the monkey. But if he also divides that pile into 5 equal parts and throws the remaining coconut to the monkey, then \(C_1=5S_2+1\) also holds, and so on. Writing down all six of these equations describing the division of coconuts at each stage:
\[\begin{align*}
C_0&=1+5S_1\\
C_1=4S_1&=5S_2+1\\
C_2=4S_2&=5S_3+1\qquad\qquad(1)\\
C_3=4S_3&=5S_4+1\\
C_4=4S_4&=5S_5+1\\
C_5=4S_5&=5S_6+1
\end{align*}
\]
Oh great, six equations and twelve unknows?! Please bear with me a little longer. With the equations in (1), we can try to express five of the \(S_i\)'s in function of their sucessors \(S_{i+1}\):
\[\begin{align*}
S_1&=\frac{1+5S_2}{4}\\
S_2&=\frac{1+5S_3}{4}\\
S_3&=\frac{1+5S_4}{4}\qquad\qquad(2)\\
S_4&=\frac{1+5S_5}{4}\\
S_5&=\frac{1+5S_6}{4}
\end{align*}\]
Some backward substitution, starting with the last two equations in (2) and working on up to the top equation there allows us to express \(S_1\) in terms of \(S_6\) and substituting this in the first equation of (1), we get:
\[ C_0=\frac{5}{4}\left(\frac{5}{4}\left(\frac{5}{4}\left(\frac{5}{4}\left(\frac{5}{4}\left(5S_6+1\right)+1\right)+1\right)+1\right)+1\right)+1\qquad(3)
\]
Working with this last equation:
\[
C_0=\frac{5^6}{4^5}S_6+\sum_{i=0}^5 \left(\frac{5}{4}\right)^i\qquad(4)
\]
In the right hand side of (4) we have a geometric series so we apply the pertinent formula:
\[ C_0=\frac{5^6}{4^5}S_6+\frac{\left(\tfrac{5}{4}\right)^6-1}{\tfrac{5}{4}-1}\qquad(5)
\]
This means that:
\[\begin{align*}
C_0&=\frac{5^6}{4^5}-4+\frac{5^6}{4^5}S_6\quad\rightarrow\\
4^5C_0&=5^6-4^6+5^6S_6\quad\rightarrow\quad&(5a)\\
1024C_0&-15625S_6=11529\qquad\quad&(5b)
\end{align*}\]
Equation (5b), which looks just like an innocent linear equation on two variables, is called a linear diophantine equation. If \(C_0\) and \(S_6\) were allowed to assume real values, we simply would have infinite solutions, but there's a catch here: we're looking for integer values of \(C_0\) and \(S_6\) as solutions to (5b). This is typical of diophantine problems, which usually involve less equations than unknowns but in which integer solutions are required.
What comes next is a little sleight of hand. Due to the symmetry in equation (5a), we can see that if \(C_0\) and \(S_6\) are one solution to the equation, so are \(C_0'=C_0+5^6\) and \(S_6'=S_6+4^5\). If we substite \(C_0'\) for \(C_0\) and \(S_6'\) for \(S_6\) in equation (5a) and notice that the \(4^55^6\) terms cancel out on both sides of the equality, the resulting equation is essentially the same:
\[
4^5(C_0+5^6)=5^6(S_6+4^5)+5^6-4^6
\]
What this all amounts to is that we can find other solutions by adding some constants to each of the variables in a known solution pair. But, how do we find one solution pair? The trick is to add \(4^6\) to both sides of (5a) and to factor out both sides:
\[
4^5(C_0+4)=5^6(S_6+1)\qquad(6)
\]
Now this last equation is easy to solve. We just need to make both sides of (6) equal to zero and obtain \(C_0=-4\) and \(S_6=-1\). But the problem is, how can we have negative coconuts?4 This is not really a problem because if we add \(5^6\) to \(C_0\) and \(4^5\) to \(S_6\), we know we can get another solution pair just as mathematically valid, but more sensible, given the problem. We finally get \(C_0=15625-4=15621\) and \(S_6=1024-1=1023\), which concur with the solutions we obtained by brute-force.
Taking a crack at diophantine equations with R
We're not about to give up on trying to find a more efficient and possibly vectorized way to solve this problem using R. I looked for an R package to solve diophantine equations and found that the "elliptic" package has a function called "congruence" which deals with linear diophantine equations of the type \(mx+by=l\).
To be honest, I could never get this function to give me the solution to equation (5b). Poring through the package's vignette, I encountered some frightening number theoretic stuff, so given my ignorance and aversion to number theory5, I gave up on using this package but before doing so, I inspected the R code for the congruence function:
1 2 3 4 5 6 7 8 9 10 11 12 13 14 15 16 17 18 19 20 21 22 23 24 25 26 27 28 29 30 31 32 |
|
Hmmm, the code above doesn't look so inscrutable. I mean, it uses the expected integer modulo operator in R (the %%'s in the code). Maybe if I knew a little bit more about diophantine equations I could reimplement this function from scratch and get it to work. So I looked up the Wikipedia article on diophantine equations and found the following theorem:
The linear diophantine equation \(ax+by=c\), where \(a\),\(b\),\(c\),\(x\) and \(y\) are integers, has a solution if and only if \(c\) is a multiple of the greatest common divisor of \(a\) and \(b\). Moreover, if \((x, y)\) is a solution, then the other solutions have the form \((x + kv,y − ku)\), where \(k\) is an arbitrary integer, and \(u\) and \(v\) are the quotients of \(a\) and \(b\) (respectively) by the greatest common divisor of \(a\) and \(b\).
This theorem is really handy for two reasons: (1) it gives us the conditions for the existence of solutions to the diophantine equation and (2) it gives us a mechanism for generating infinitely more solutions if we are able to find one solution. This last point, finding one solution, is what I would have to tackle in my implementation of the code.
I decided that I would look for a first solution by separating each variable to one side of the \(ax+by=c\) equation and getting \(ax=c-by\) or \(by=c-ax\). Then, taking \(ax=c-by\) for example, I would have my code try out a range of values for \(y\) to verify when the expression \(c-by\) is divisible by \(a\). Intuitively, I realized that the range of values to try for \(y\) would be all integers between 0 and \(a\) (when using \(by=c-ax\), I would have to try all integers between 0 and \(b\) for \(x\)), so it's not like I would have to search through an infinitely large solution space.
Having found values of \(x\) or \(y\), which are guaranteed to exist if the solution existence conditions in the Wikipedia theorem are met, I would substitute this value in the equation to obtain the other value. Finally, I would have to implement a function for finding the greatest common divisor. All in all, I came up with the following code:
1 2 3 4 5 6 7 8 9 10 11 12 13 14 15 16 17 18 19 20 21 22 23 24 25 26 27 28 29 30 |
|
## [,1] [,2]
## [1,] 15621 1023
## [2,] -15625 -1024
As you can see, it works like a charm. This is a code I can be proud of (look ma, no for/while loops!). My function not only gives me the solution in the first row of the results matrix, but gives me the coefficients whose multiples I would have to add to \(x\) and \(y\) to get other solution pairs. I haven't really proven that it works for all possible values of m, n and l, but I tried a few example problems which I solved by hand and it seems to be ok. Needless to say, I invite my readers to put this code under close scrutiny (you know what they say about many pairs of eyes seeing more than one). Perhaps some of you will find errors in the code, or a test-case which "breaks" the code. Also, I invite my readers to come up with an R function for solving general 3 or more variable linear diophantine equations. For further discussion or questions of this, I invite you to participate in the comments for this post.
Notes
- I guess I got carried away with that pitchfork and torch bearing angry mob bit, but I do wish we Venezuelans would organize into such a mob to evict Maduro from power. Ironically, it seems like in the days when there were no social networks or smartphones, people somehow organized into action more effectively - for example during the 1958 ousting of the Perez-Jiménez dictatorship. Now, we somehow expect the Internet to "do things for us", while we stay at home.
- When teaching math, it is never a good idea to just go ahead and show students the "clean" mathematical solution. Teaching math is all about modeling the problem-solving process, which in real life, is usually a messy, trial-and-error roundabout affair. When we have become experts in making mistakes and following dead-end leads, we will have gained sufficient familiarity with the problem and only then can we hope to come up with a clean solution.
- R is not particularly fast when running for/while loops. What R programmers always strive to do is to vectorize their code. Vectorization consists of avoiding for/while loops all together and working with vectors and functions of vectors. In the next script, we will be using vectorized code. So you could say that in R, using for or while loops in brute-search is the most brutish way to go about it.
- I wonder what negative coconuts taste like...
- My apologies to number-theorists (they would cringe at a blog like this anyways), but number theory seems to me like the most useless branch of mathematics. I mean, of what practical use is Fermat's Last Theorem or Goldbach's Conjecture? Sure, probably the most difficult and even unresolved or unproven math problems are buried deep within number theory. Sure, solving these problems would be an instant tiket to mathematical fame and fortune - the stuff of legends. As for me, I prefer to steer clear of things for which I just don't have the talent, so that I won't suffer the same fate as Uncle Petros. Uncle Petros, by the way, is the main character in an extremely entertaining novel about mathematics by Apostolos Doxiadis, called Uncle Petros and Goldbach's Conjecture
Bibliography
- Diophantine equation. (2016, July 26). In Wikipedia, The Free Encyclopedia. Retrieved September 4, 2016, from https://en.wikipedia.org/wiki/Diophantine_equation
- HANKIN, R. K. S. (February 2006). Introducing Elliptic, an R package for Elliptic and Modular Functions. Journal of Statistical Software, Volume 15, Issue 7.
- PADILLA, T. and McPARTLAN, P. [Numberphile] (2015, April 29). Monkeys and Coconuts - Numberphile [Video file]. Retrieved September 4, 2016, from https://www.youtube.com/watch?v=U9qU20VmvaU.
- R DEVELOPMENT CORE TEAM (2009). R: A Language and Environment for Statistical Computing. R Foundation for Statistical Computing, Vienna, Austria. ISBN 3-900051-07-0. http://www.R-project.org.
If you found this post interesting or useful, please share it on Google+, Facebook or Twitter so that others may find it too.